В современном динамичном мире бизнеса финансовая грамотность является не просто желательной компетенцией, но и жизненно важным навыком для успешной деятельности.
Особенно это актуально для семейных предприятий, где каждое решение может повлиять не только на бизнес, но и на взаимоотношения внутри семьи.
Групповые сессии по финансовому планированию предоставляют уникальную возможность не только повысить уровень знаний всей команды, но и создать общее понимание стратегии и целей компании. Изучая основы финансов, участники этих сессий могут лучше понять природу рисков, возникающих перед предприятием, и возможные пути их минимизации. Открытое обсуждение финансовых вопросов в группе также способствует формированию культуры прозрачности и взаимного доверия. В этой статье мы рассмотрим, почему групповые сессии так важны, какие темы стоит затронуть и как правильно организовать этот процесс.
Почему групповые сессии эффективны?
Групповой формат обучения имеет ряд преимуществ перед индивидуальным:
- Взаимодействие и обмен опытом. Участники могут делиться своими знаниями, ошибками и успехами, что ускоряет процесс обучения.
- Групповая динамика. Работая в команде, люди становятся более мотивированными, что способствует лучшему усвоению материала.
- Снижение стоимости обучения. Организация групповых сессий часто обходится дешевле, чем индивидуальные консультации или курсы.
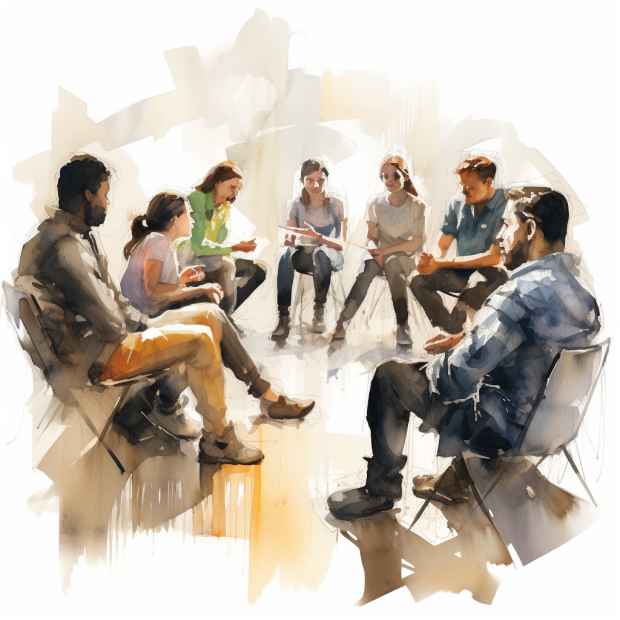
Основные темы для обсуждения на групповых сессиях
Качественное и плодотворное обсуждение на групповых сессиях требует чёткого понимания ключевых тем и вопросов, которые будут на повестке дня. Давайте более подробно рассмотрим основные направления, которые стоит затронуть во время таких мероприятий:
Базовые принципы финансового планирования:
- Основы бухгалтерии: Понимание базовых концепций, таких как активы, пассивы, доходы и расходы, является краеугольным камнем для понимания более сложных финансовых механизмов.
- Налогообложение: Осознание основных налоговых обязательств и способов оптимизации налогов может значительно повлиять на финансовое положение компании.
- Бюджетирование и прогнозирование: Разработка и следование бюджету, а также умение предсказывать будущие финансовые потоки, помогут компании поддерживать устойчивость и планировать рост.
Инвестиционные стратегии:
- Основы инвестирования: Понимание разницы между различными инвестиционными инструментами, такими как акции, облигации и взаимные фонды.
- Риски и доходность: Обсуждение принципа «больший риск — больший доход» и методов управления портфелем для максимизации дохода при минимальных рисках.
- Долгосрочное и краткосрочное инвестирование: Различия между долгосрочными и краткосрочными стратегиями и их применение в контексте семейного бизнеса.
Управление рисками:
- Типы финансовых рисков: От рыночных и кредитных рисков до операционных и репутационных рисков.
- Инструменты и методы управления рисками: Применение деривативов, страхования и диверсификации для снижения потенциальных убытков.
- Создание культуры управления рисками: Внедрение процедур и протоколов для обеспечения прозрачности и принятия информированных решений на всех уровнях организации.
Рассмотрение этих тем на групповых сессиях поможет создать общий фундамент знаний среди членов команды и усилит единство внутри коллектива в плане финансового планирования.
Организация и подготовка к групповым сессиям
Чтобы сессия прошла наиболее эффективно, рекомендуется:
- Подготовить материалы. Презентации, видео, интерактивные задания — все, что поможет участникам лучше усвоить информацию.
- Пригласить эксперта. Если в вашей команде нет специалиста по финансам, стоит пригласить внешнего консультанта.
- Создать комфортную атмосферу. Удобное помещение, кофе-брейк и непринужденная обстановка помогут участникам сконцентрироваться на процессе обучения.
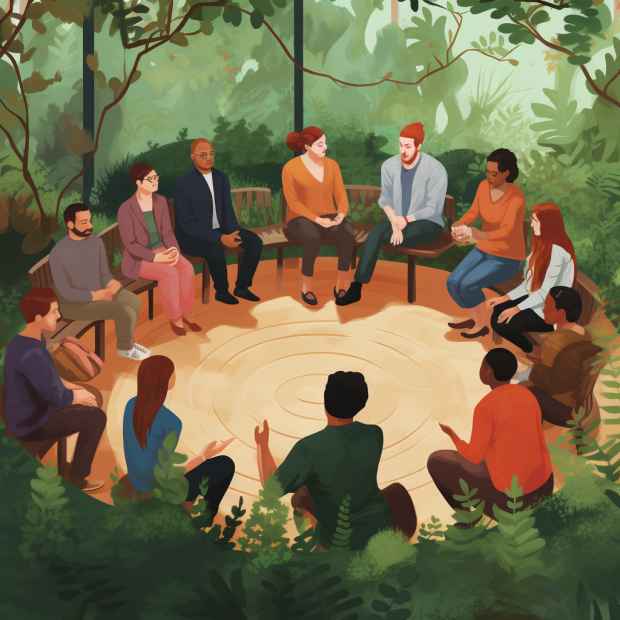
Заключение
Групповые сессии по финансовому планированию — отличный способ повысить квалификацию вашей команды, улучшить взаимодействие в коллективе и, в конечном итоге, повысить эффективность вашего бизнеса.
Вопросы и ответы:
Да, современные инструменты для видеоконференций позволяют организовать качественные сессии дистанционно.
В среднем, одна сессия должна длиться от 1 до 3 часов. Слишком длинные занятия могут снизить концентрацию участников.
Это зависит от потребностей вашей команды. Начните с ежемесячных сессий и, по мере необходимости, корректируйте график.